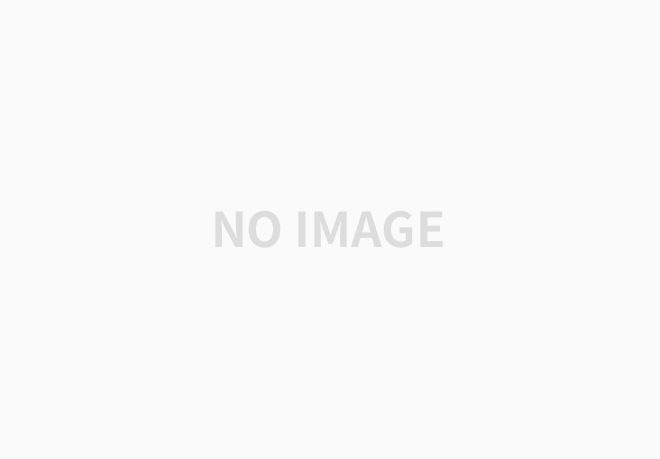
Information
- DCCN (Dilated Causal Convolution Neural Network) is a deep learning architecture used for time series forecasting that combines dilated causal convolutional layers and residual connections.
- Dilated causal convolutions are a variant of convolutional layers that incorporate time information and can capture long-term dependencies in time series data.
- The causal aspect of the convolutions ensures that the output at each time step only depends on past inputs and not future ones, making it suitable for forecasting tasks.
- Residual connections are used to connect the input and output of a convolutional block, allowing the network to learn residual mappings from the input to the output, which helps to prevent vanishing gradients during training.
- DCCN has been shown to outperform other state-of-the-art methods for time series forecasting tasks, such as ARIMA and LSTM, on a variety of datasets.
- DCCN has been applied to a range of energy-related problems such as load forecasting, wind power prediction, and solar power prediction.
- DCCN has the advantage of being computationally efficient, making it a suitable option for real-time forecasting applications.
'Zettelkasten > Terminology Information' 카테고리의 다른 글
BiLSTM (Bidirectional Long Short-Term Memory) (0) | 2023.03.13 |
---|---|
P-value (0) | 2023.03.13 |
CART (Classification And Regression Tree) (0) | 2023.03.12 |
DL (Deep Learning) (0) | 2023.03.11 |
Regression tree (0) | 2023.03.11 |
댓글