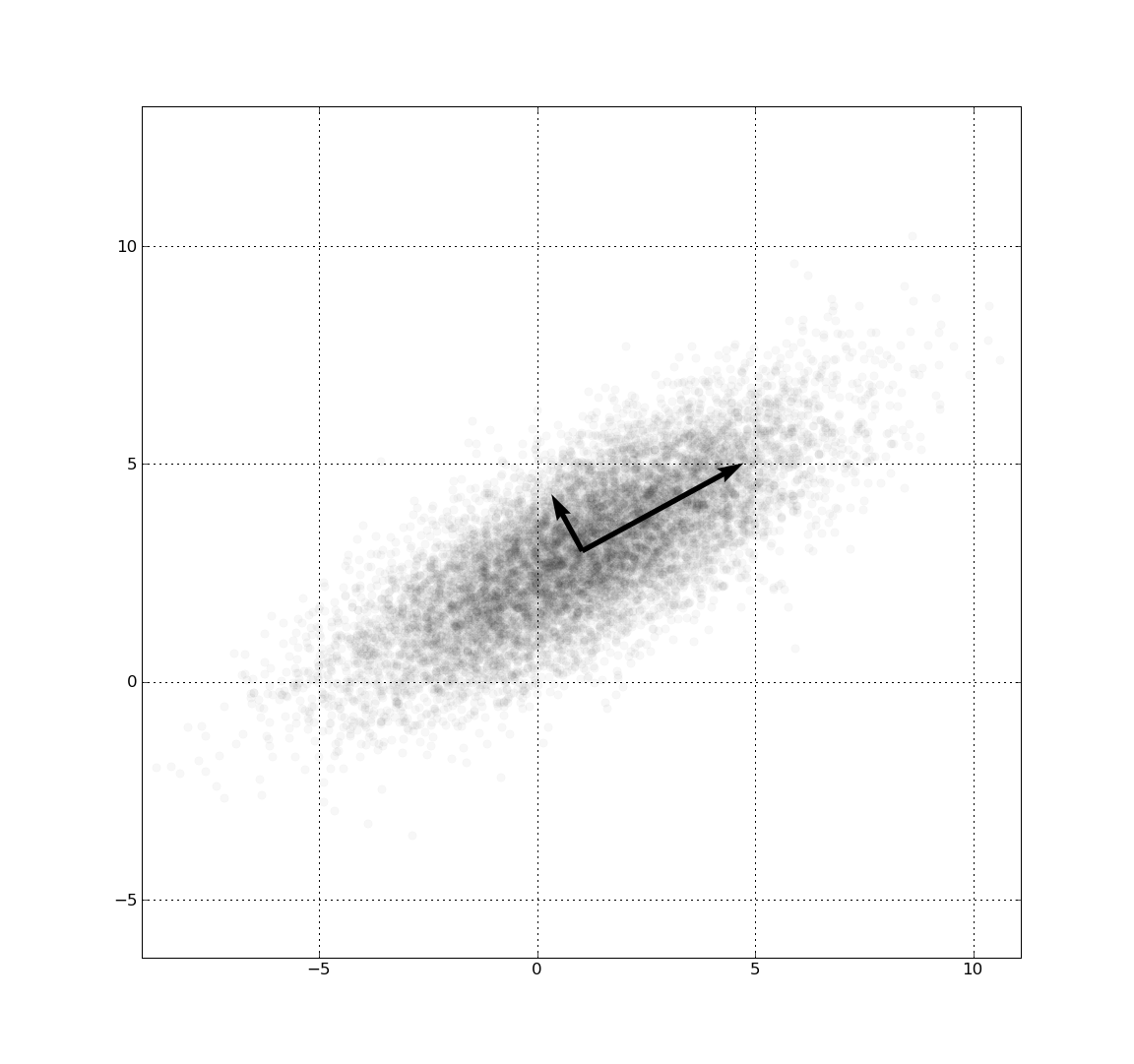
Information
- PCA (Principal Component Analysis) is a statistical technique used to reduce the dimensionality of large datasets, while retaining as much of the variance or information as possible.
- PCA works by transforming the original variables into a new set of orthogonal variables, called principal components, that explain the maximum variance of the data, with the first component explaining the largest variance, the second component explaining the second largest variance, and so on.
- PCA assumes that the data is normally distributed and linearly correlated, and that the variables are standardized or scaled to have zero mean and unit variance, in order to avoid bias and numerical instabilities.
- PCA can be applied to various types of data, such as continuous or discrete variables, numeric or categorical variables, and even images or signals, by defining appropriate similarity or covariance matrices.
- PCA can be used for various purposes, such as data compression, visualization, feature extraction, and anomaly detection, by selecting a subset of the principal components that capture the most relevant information for a given task, while discarding the noise or redundancy.
- PCA can be combined with other techniques, such as clustering, classification, or regression, to improve their performance, by reducing the dimensionality and improving the interpretability of the data.
- PCA has several limitations, such as the sensitivity to outliers or nonlinearity, the loss of interpretability of the original variables, the requirement of large sample sizes, and the difficulty of selecting the optimal number of principal components.
'Zettelkasten > Terminology Information' 카테고리의 다른 글
ML (Machine Learning) (0) | 2023.03.03 |
---|---|
Persistence (0) | 2023.03.03 |
MLR (Multiple Linear Regression) (0) | 2023.03.02 |
STLF (Short-Term Load Forecasting) (0) | 2023.03.01 |
ES (Exponential Smoothing) (0) | 2023.03.01 |
댓글